How Predictive Analytics is Transforming Modern Enterprises
- Quixom
- Apr 14
- 5 min read
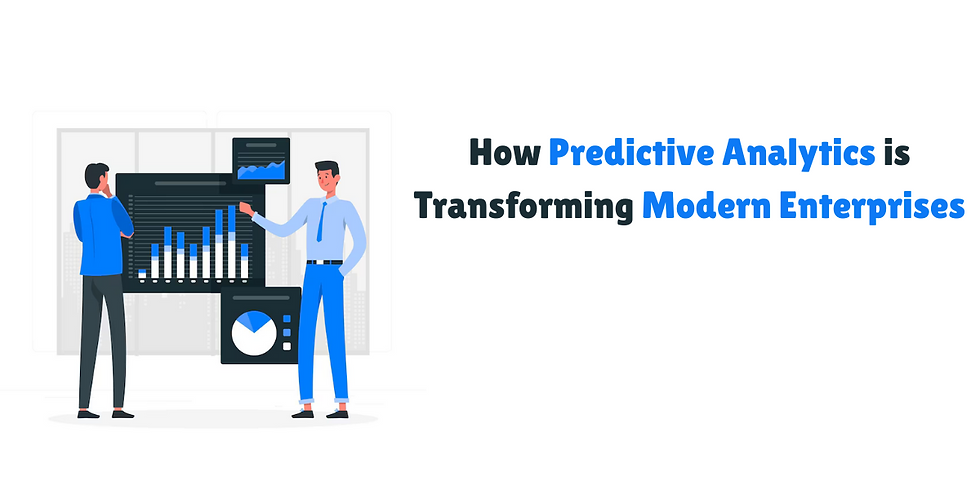
Introduction
In an era where data-driven decisions define business success, predictive analytics is revolutionizing how enterprises operate. Consider this: companies that adopt data-driven strategies are five times more likely to make faster decisions than their competitors. Netflix, for example, saved $1 billion in customer retention by using predictive analytics to suggest content based on user behavior. This level of foresight isn’t magic—it’s the result of combining data engineering, business intelligence, and forecasting using AI.
Predictive analytics involves using statistical techniques, machine learning algorithms, and data modeling to forecast future outcomes. It empowers organizations to move from reactive to proactive decision-making. By analyzing historical data and identifying patterns, predictive analytics helps businesses anticipate customer behavior, optimize operations, and mitigate risks.
In the context of modern enterprises, predictive analytics is no longer optional—it’s a strategic imperative. From supply chain optimization to personalized marketing and real-time fraud detection, the use cases are endless. Enterprises that adopt a modern data stack—integrating data migration, visualization, and engineering—are leading the way in building intelligent, scalable systems. Data analytics has become the cornerstone of digital transformation, enabling businesses to align strategy with future insights rather than past performance.
How Predictive Analytics is Transforming Modern Enterprises
Predictive analytics is fundamentally reshaping how enterprises approach strategy, operations, and customer engagement. Businesses are leveraging it to reduce uncertainty and improve forecasting accuracy across departments. In sales and marketing, predictive models are being used to identify high-converting leads and anticipate customer churn, enabling teams to act with precision. For operations, it means optimized inventory levels, reduced downtime through predictive maintenance, and improved resource allocation.
In finance, Data analytics enables more accurate revenue forecasting and risk management, offering a clearer picture of what’s ahead. Healthcare providers are using it to predict patient outcomes and tailor treatments, while retail businesses forecast product demand and personalize the shopping experience. The power lies in the ability to transform raw data into actionable insights.
What sets Data analytics apart is its adaptability across sectors. Whether you’re a facility manager optimizing energy use or a corporate real estate executive planning occupancy levels, predictive tools turn historical data into foresight. Enterprises are no longer waiting for trends to happen—they are predicting them, and pivoting accordingly. With AI-driven forecasting and real-time analysis, predictive analytics is giving businesses the agility to lead in increasingly competitive markets.
The Role of Data Engineering in Predictive Analytics
Data engineering is the unsung hero behind successful predictive analytics initiatives. It provides the infrastructure and processes necessary to collect, clean, and structure data so it can be effectively used for analysis and modeling. Without solid data pipelines and architecture, even the most sophisticated algorithms will fail to deliver accurate predictions.
Modern enterprises deal with enormous volumes of structured and unstructured data from various sources—CRMs, IoT devices, ERP systems, and more. Data engineering ensures that this information is ingested through well-designed pipelines, stored in accessible formats, and made available in real-time or near-real-time for analytics teams. It enforces data quality, eliminates duplicates, handles missing values, and transforms raw data into usable formats.
Furthermore, the rise of the modern data stack—featuring tools like Snowflake, dbt, and Apache Airflow—has enabled more scalable, efficient data engineering practices. These tools allow engineers to automate workflows, improve data lineage, and ensure robust governance. The result is a clean, reliable data foundation that supports predictive modeling and AI-based forecasting. Simply put, without data engineering, predictive analytics cannot function with the speed, scale, or accuracy that modern enterprises demand.
Data Migration: Moving Towards Predictive Infrastructure
For many enterprises, outdated legacy systems are a major roadblock to implementing Data analytics. Data migration—the process of transferring data from old systems to modern environments like cloud or hybrid architectures—is critical for enabling real-time, scalable analytics.
Cloud platforms offer powerful compute capabilities and storage solutions that can handle large-scale data processing and machine learning workloads. Migrating to these platforms allows enterprises to centralize data, eliminate silos, and enable seamless integration with advanced Data analytics tools. Hybrid solutions also offer flexibility, letting organizations manage sensitive data on-premises while utilizing cloud-based AI for Data analytics.
However, data migration is more than a technical upgrade—it’s a strategic shift. When done correctly, it improves data accessibility, enhances security, and supports business intelligence initiatives. A well-planned migration ensures data consistency, integrity, and availability, all of which are essential for successful predictive analytics. By investing in migration, enterprises position themselves for future growth, unlocking the full potential of their data assets.
Technologies Powering Predictive Analytics
The engine behind predictive analytics includes a suite of powerful technologies that work in synergy. Machine learning algorithms, both supervised and unsupervised, are at the core. These algorithms analyze past data to make predictions about future outcomes. Techniques like regression analysis, decision trees, and neural networks are commonly used.
AI-driven forecasting tools are enhancing predictive capabilities further, offering more accurate and faster results. Platforms like AWS SageMaker, Google Cloud AI, and Azure Machine Learning simplify model development and deployment at scale. Meanwhile, data visualization tools such as Power BI, Tableau, and Looker play a vital role in making insights understandable and actionable for stakeholders.
In addition, real-time analytics powered by in-memory computing (like Apache Kafka and Spark) allows businesses to act on insights instantly. Integration with IoT devices, edge computing, and streaming data sources enables dynamic predictive models that evolve with changing inputs. Combined with robust data engineering, these technologies provide a complete ecosystem that supports enterprise-wide predictive analytics.
The Future of Predictive Analytics in Enterprises
The future of predictive analytics is both exciting and transformative. With AutoML (automated machine learning) becoming more accessible, enterprises can build and deploy models without requiring deep data science expertise. This democratization allows more departments to leverage predictive insights.
Real-time analytics will become the norm, enabling businesses to make split-second decisions based on live data. The integration of generative AI is already reshaping how models are trained, providing synthetic data for better model accuracy. Moreover, predictive analytics combined with IoT and edge computing is driving innovation in areas like smart buildings, autonomous operations, and energy efficiency.
As technology evolves, enterprises must prepare for a predictive-first strategy. This involves investing in a modern data stack, upskilling teams, and redefining KPIs to be more forward-looking. The convergence of predictive analytics with business intelligence will create smarter, more agile organizations. For facility managers, CIOs, and property professionals, now is the time to embrace predictive transformation or risk falling behind.
Conclusion
Predictive analytics is no longer a futuristic concept—it’s a present-day necessity. It empowers enterprises to anticipate change, reduce risk, and identify opportunities with precision. By integrating technologies like machine learning, AI forecasting, and real-time analytics, businesses can transition from reactive to proactive decision-making. Whether you're managing physical assets, optimizing energy consumption, or improving customer retention, Data analytics offers a competitive edge.
But success in this space requires more than just algorithms. It starts with strong data engineering, ensuring your data is clean, accessible, and reliable. It involves thoughtful data migration to scalable cloud environments, laying the groundwork for real-time insights. And it demands visualization tools that translate complex models into business value.
For facility managers, CIOs, and operations leaders, the message is clear: predictive analytics isn't just about technology—it's about transformation. It aligns operations with future demand, enhances efficiency, and delivers measurable ROI. As enterprises face increasing complexity and competition, those who adopt predictive strategies will not only survive—they will lead.
Comments